Research
Traditionally, increased speed and lower power consumption in modern electronics has been achieved through aggressive transistor scaling and more elegant architectural designs. Another pathway that has been explored extensively in the recent years is the use of new computational devices. ReRAM (also considered as a category of "memristors") are novel two terminal electronic devices based on resistive switching, a physical phenomena where a dielectric rapidly changes its resistance under strong applied voltage. These devices can be used in a variety of data storage, processing and communication applications, as highlighted in the recent projects below.
Our research is kindly supported by external funding agencies and by intramural grants from George Washington University.
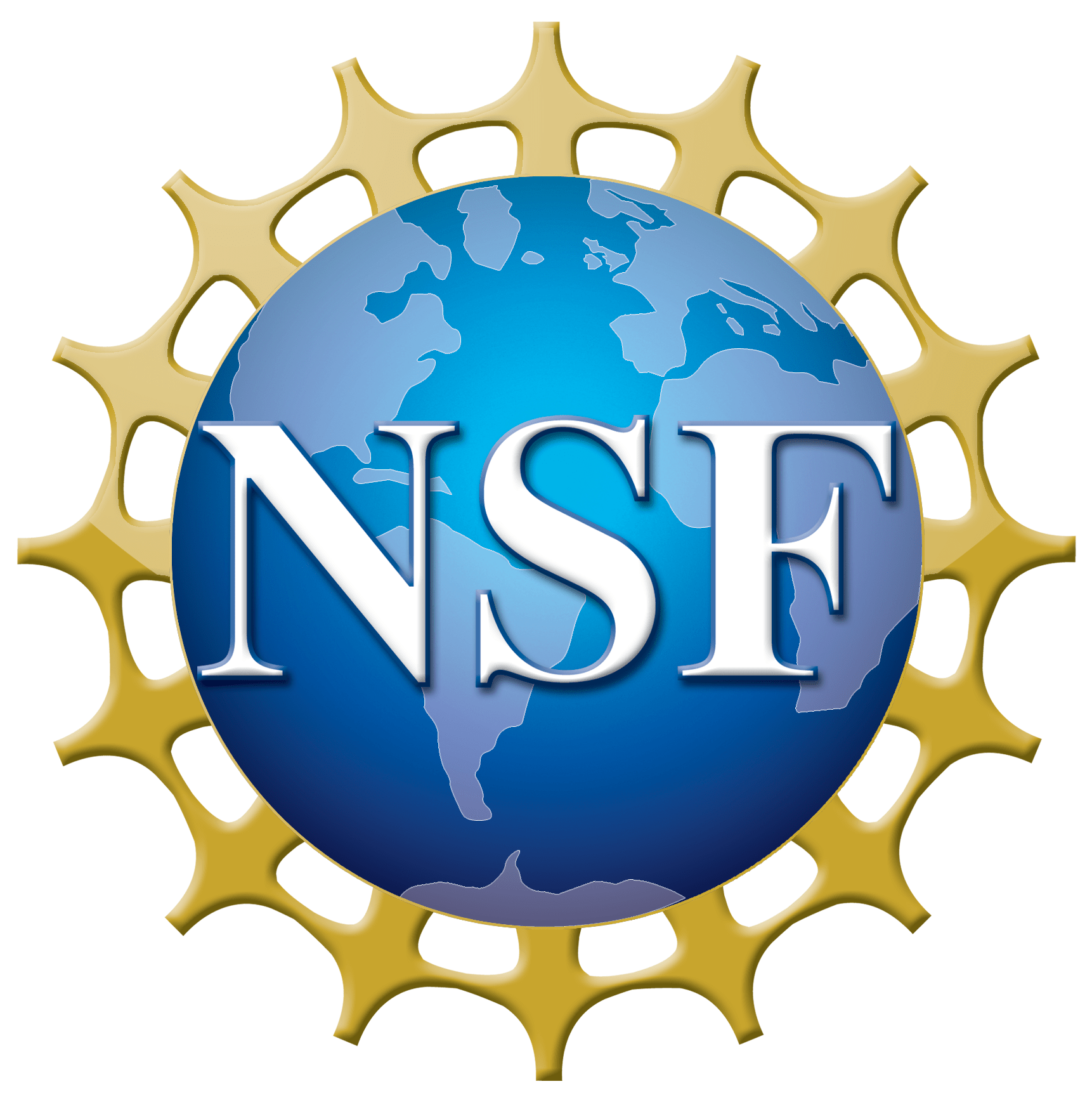
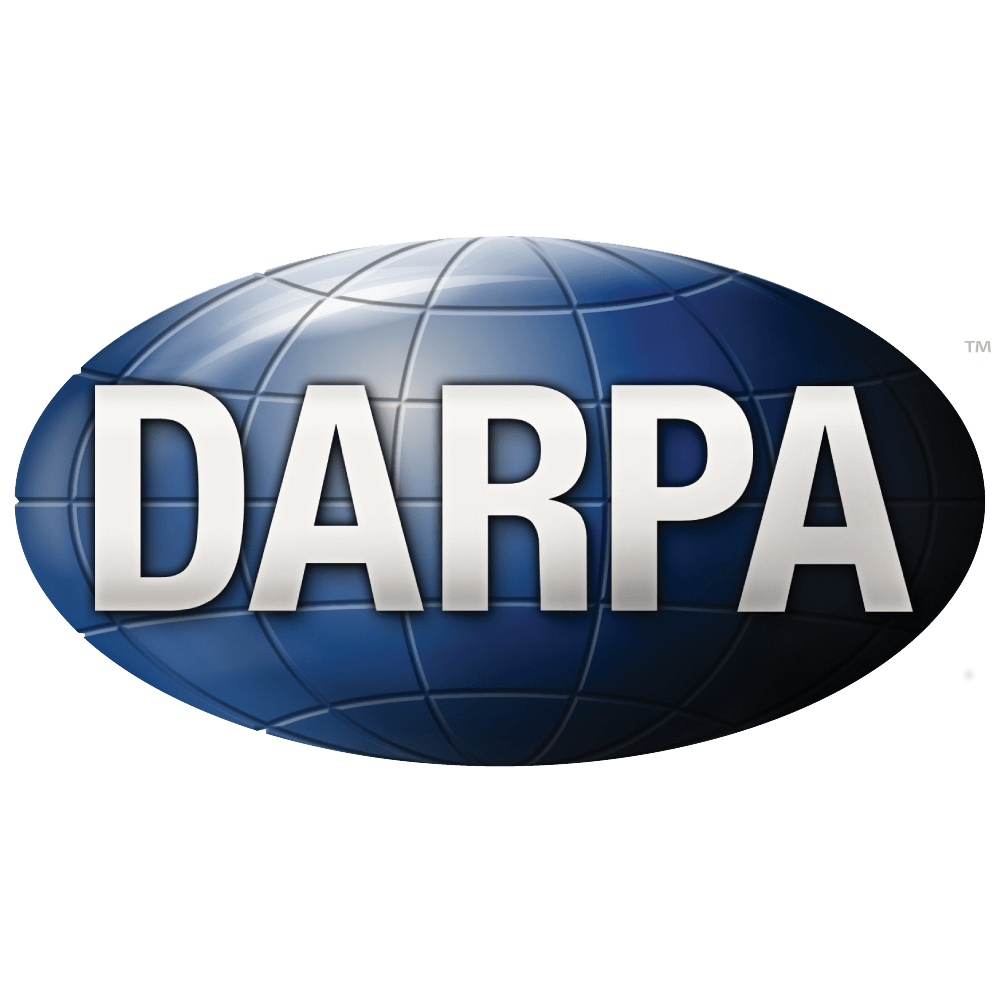
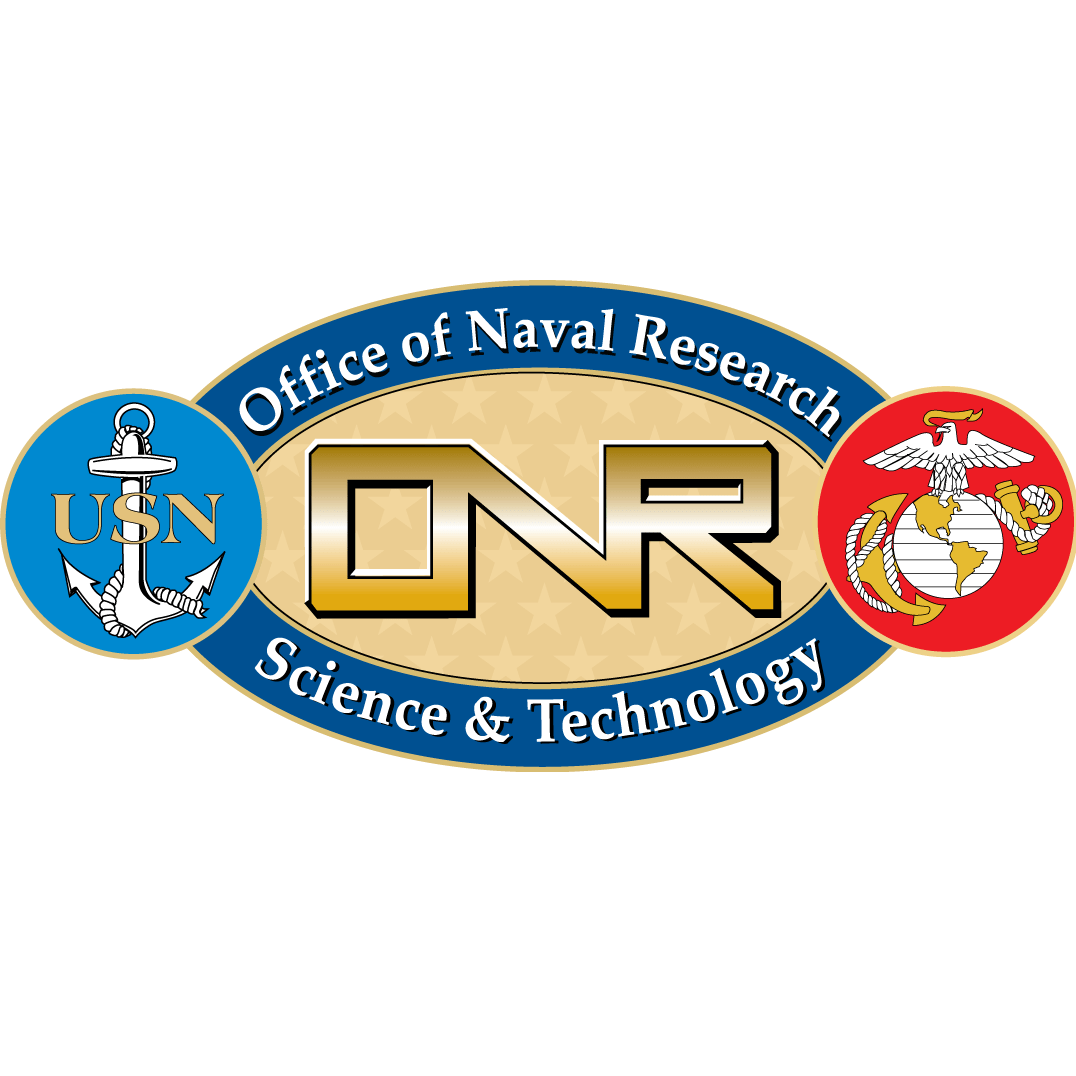
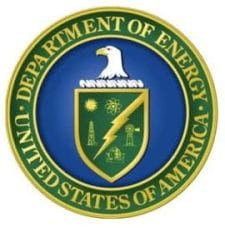
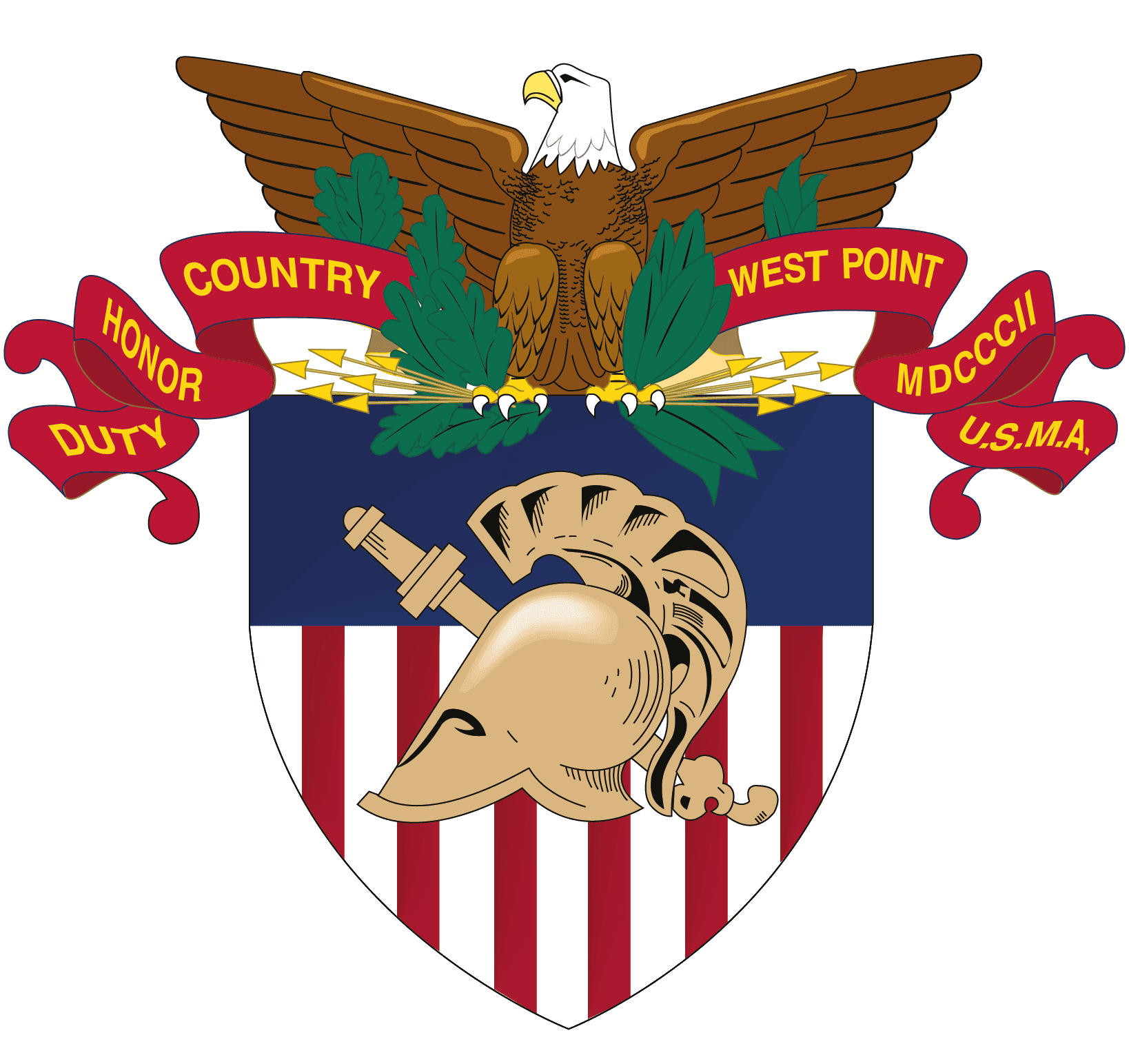
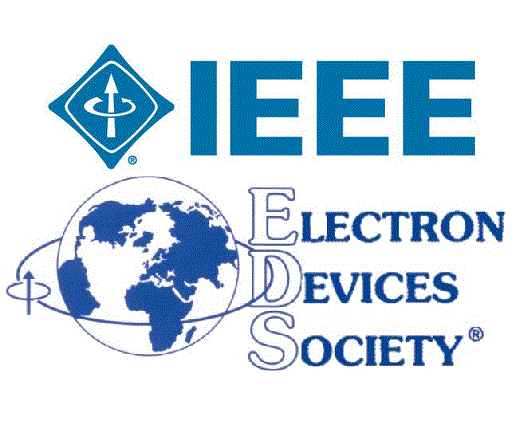
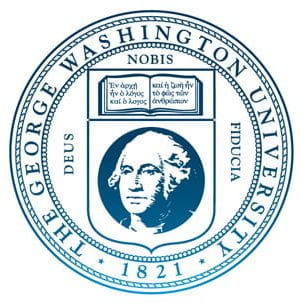
Memristor fabrication and integration
A main goal of our work is to integrate ReRAM switches, emerging two-terminal devices with interesting analog and digital behaviors, into arrays and dense matrices (crossbars) and stack them in three-dimensions for ultra-dense storage and computing. For this endeavour, we benefit from the state-of-the-art facilities at the GW Nanofabrication and Imaging Center.
An important challenge for this technology is the device variability in operation, due to the fact that these devices are based on little explored amorphous metal oxides rather than on the traditional crystalline silicon. We aim to engineer the ReRAM devices by investigating their device physics using non-invasive methods and engineering their material stack in order to achieve desired low operating voltages and high device uniformity and yield. Previous work allowed us to integrate these devices into standalone dense ReRAM crossbars [1,3] but also onto traditional CMOS chips [2] and further use these circuits in various applications, such as digital implication logic and analog dot product. Our future efforts will focus on new types of materials and device designs, such as vertical ReRAM, in order achieve low variability and cost-effective manufacturing.
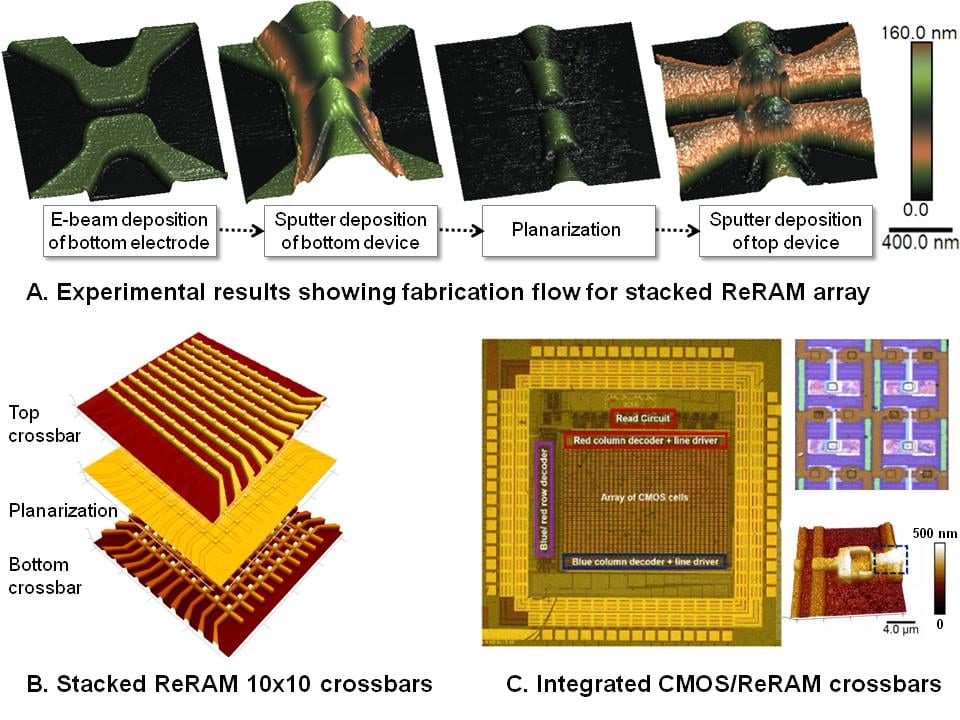
[1] G. C. Adam, B.D. Hoskins, M. Prezioso, F. Merrikh-Bayat, B. Chakrabarti, D.B. Strukov “Three-Dimensional Memristor Crossbars for Analog and Neuromorphic Computing Applications”, IEEE Transactions on Electron Devices, 64 (1), 312-318, 2017. [doi]
[2] B. Chakrabarti, M.A. Lastras-Montaño, G.C. Adam, M. Prezioso, B. Hoskins, K-T. Cheng, D.B. Strukov “A multiply-add engine with monolithically integrated 3D memristor crossbar/CMOS hybrid circuit”, Scientific Reports, 7, 42429, 2017. [doi]
[3] G. C. Adam, B.D. Hoskins, M. Prezioso, D.B. Strukov “Optimized Stateful Material Implication Logic for 3D Data Manipulation”, Nano Research, 9: (3914), 2016. [doi]
Physics of memristive switching
Resistive switches (or ReRAM or memristors), although of high interest to the academic and industry community, have a multi-physics mode of operation that makes their study difficult.
We utilize non-invasive high resolution characterization techniques to image the filament dynamic behavior as the device changes state. In a recent work performed in collaboration with McClelland group at NIST Gaithersbug [4], energy dependent and stateful EBIC measurements were performed on conventional parallel plate capacitor resistive switching devices. By exploring variations in the generated signal across shifts in geometry, beam energy, and resistive state, it was possible to observe two different scaling relationships for the turn-on and turn-off of the switching, suggesting different mechanisms of filament formation and dissolution. Our future work will utilize this technique and other similar to explore how the details of device manufacturing and geometry impact the scaling of the resistive switching.
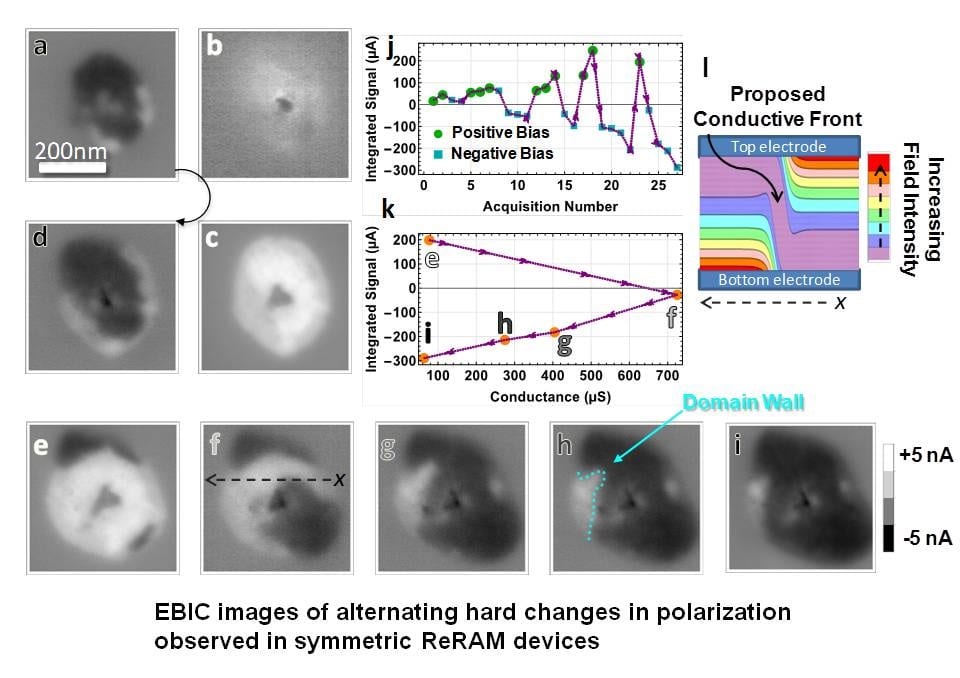
[4] B.D. Hoskins, G. C. Adam, E. Strelcov, N. Zhitenev, A. Kolmakov, D.B. Strukov, J. J. McClelland. “Stateful characterization of resistive switching TiO2 with electron beam induced currents”, Nature Communications, 1972, 2017 [doi]
Memristive neuromorphic computing
Neuromorphic computing systems, electronic systems that try to mimic the functionality of the biological brain, promise to deliver faster and more energy efficient computation in areas like processing of visual information or natural language where today’s computers perform poorly. By comparison with the current hardware technology based on digital (either “0” or “1”) silicon transistors, the ReRAM devices are analog, with multiple intermediate states, similar to an artificial which shows great promise for this field. A matrix of ReRAM devices can implement naturally through Ohm’s law the otherwise resource intensive vector-matrix multiplication – a crucial part in artificial neural networks. Previous work showed how a 12x12 matrix of such devices was trained to implement the simplest neuromorphich circuit, a single layer feed-forward perceptron. This implementation showed 100% classification of 30 patterns representing three letters and their noisy version [5,6]. These ReRAM devices were also used to experimentally prove robust STDP behavior thanks to an explicit initial-state-dependent implementation [7].
Our future work will focus on ReRAM implementations of more complex networks such as recurrent neural networks (RNNs). RNNs have the advantage of information persistency over the traditional feed-forward neural networks, thus making it possible to analyze, categorize and even make predictions on sequences of signals. Their recurrent loops enable natural language processing, video classification and captioning, robot control, etc.
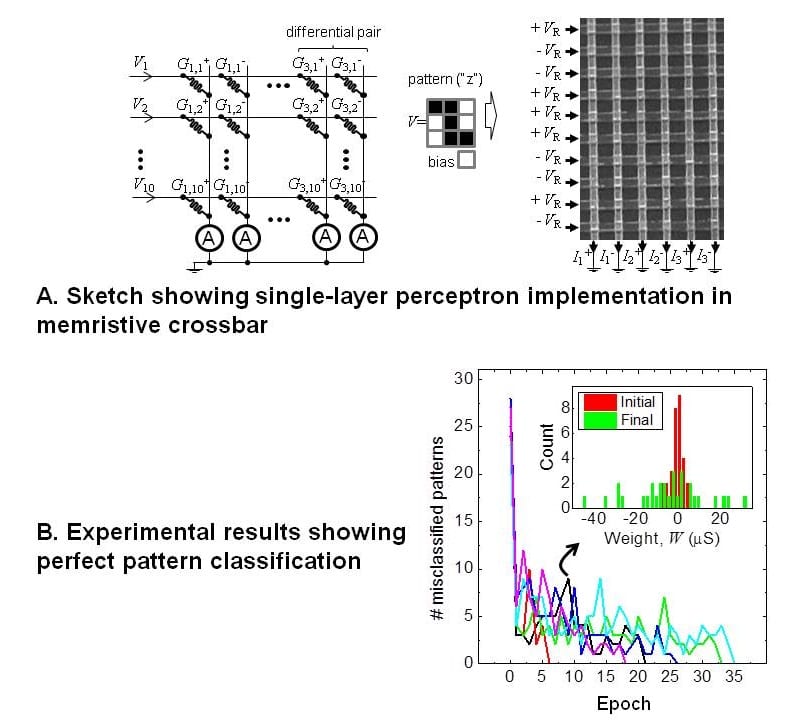
[5] Prezioso, M., Merrikh-Bayat, F., Hoskins, B. D., Adam, G. C., Likharev, K. K., & Strukov, D. B. "Training and operation of an integrated neuromorphic network based on metal-oxide memristors". Nature, 521(7550), 61-64, 2015.[doi]
[6] Prezioso, M., I. Kataeva, F. Merrikh-Bayat, B. Hoskins, G. Adam, T. Sota, K. Likharev, and D. Strukov. "Modeling and implementation of firing-rate neuromorphic-network classifiers with bilayer Pt/Al2O3/TiO2− x/Pt Memristors." In Electron Devices Meeting (IEDM), 2015 IEEE International, pp. 17-4. IEEE, 2015. [doi]
[7] Prezioso, M., Y. Zhong, D. Gavrilov, F. Merrikh-Bayat, B. Hoskins, G. Adam, K. Likharev, and D. Strukov. "Spiking neuromorphic networks with metal-oxide memristors." In Circuits and Systems (ISCAS), 2016 IEEE International Symposium on, pp. 177-180. IEEE, 2016. [doi]